Python Inception
At most companies I have worked for, there was some internal Python code base that relied on an old version of Python. But especially for data science, I'd often want to execute that code from an up-to-date Jupyter Notebook, to do some analysis on results.
When this happened last time, I decided to do something about it. Here's a Jupyter cell magic that executes the cell's code in a different Python, pipes out all of STDOUT and STDERR, and imports any newly created variables into the host Python. Use it like this:
%%py_magic /old/version/of/python import this truth = 42
When this cell executes, you will see the Zen of Python in your output, just as if you had import this
in the host Python, and the variable truth
will now be 42 in the host Python.
To get this magic, execute the following code in a preceding cell:
import subprocess import sys import pickle import textwrap from IPython.core.magic import needs_local_scope, register_cell_magic @register_cell_magic @needs_local_scope def py_magic(line, cell, local_ns=None): proc = subprocess.Popen([line or 'python'], stdin=subprocess.PIPE, stdout=subprocess.PIPE, stderr=subprocess.PIPE, encoding='UTF8') # send a preamble to the client python, and remember all pre-existing local variable names: proc.stdin.write(textwrap.dedent(""" import pickle as _pickle import types as _types _names_before = [k for k, v in locals().items()] + ['_f', '_names_before'] try: """)) # send the cell's contents, indented to run in the try: for line in cell.splitlines(): proc.stdin.write(" " + line + "\n") # indent! # send a postamble that pickles all new variables or thrown exceptions: proc.stdin.write(textwrap.dedent(""" # save results to result.pickle except Exception as exc: with open('result.pickle', 'wb') as _f: _pickle.dump({'type':'error', 'value': exc}, _f) else: with open('result.pickle', 'wb') as _f: _values = {k:v for k, v in locals().items() if not isinstance(v, _types.ModuleType) and not k in _names_before} _safe_values = {} # skip any unpickleable variables for k, v in _values.items(): try: _pickle.dumps(v) except Exception as _exc: print(f'skipping dumping {k} because {_exc}') else: _safe_values[k] = v _pickle.dump({'type':'result', 'value': _safe_values}, _f) finally: quit() """)) # print any captured stdout or stderr: stdout, stderr = proc.communicate() if stdout: print(stdout, file=sys.stdout) if stderr: print(stderr, file=sys.stderr) # load new local variables or throw error: try: with open('result.pickle', 'rb') as f: result = pickle.load(f) if result['type'] == 'error': raise result['value'] elif result['type'] == 'result': for key, value in result['value'].items(): try: local_ns[key] = value except Exception as exc: print(f"skipping loading {key} because {exc}") finally: pathlib.Path('result.pickle').unlink() # remove temporary file del py_magic # otherwise the function overwrites the magic
I love how this sort of trickery is relatively easy in Python. Also, this is the first time I've used a try
with except
, else
, and finally
.
AI Predictions
Meta just invested 30 Billion Dollars into AI accelerators1. That's roughly equivalent to one Manhattan Project worth of money. Meta must expect a comparable return on that investment. But with that kind of money, that return must be disruptive.
And yet, AI does not feel disruptive. In my life, I have witnessed a few consumer technology disruptions: portable computers, portable telephones, internet-connected "smart" telephones, always-available GPS. Perhaps even tablet computers, smart watches, and electric cars? They all felt exciting! They all felt immediately obviously useful, if perhaps not always to me. But AI does not excite me. So if it's not for me, where is that $30B market that Meta is envisioning?
The best I can think of is a "command line for the common man". But the power of the command line comes from unflinchingly powerful commands, and behaving deterministically. Both of these characteristics are the antithesis of current AI technology.
We will not give an AI assistant the power to execute "format my hard drive", or "delete my Google account", even though a command line interface clearly would. Yet without that power, the AI assistant is toothless, and less useful. Even if we did, how could we trust the AI assistant to actually do what we want, and not misunderstand us? When interacting with LLMs, I am reminded of Terry Pratchett's gods of the Discworld, who wield absolute power, but you don't want to ask them for help as they're too likely to do what they think you wanted instead of what you actually asked.
But without power, and without deterministic behavior, you can't have a command line experience.
I keep coming back to that question: What is the disruptive use case of AI? Sure, we'll outsource some tasks to AI that we'd previously outsource overseas. This will result in rampant tragedy, and is already known to be difficult to pull off successfully. We'll enhance many tasks with short snippets of AI, to speed up menial programming tasks, and writing tasks, translation, image generation. But that's a feature-addition to existing software, not a disruption, let alone a $30B disruption.
Perhaps I'm wrong. Only time will tell. But I hereby predict that disruptive AI is a bubble.
Footnotes:
: For reference, one Billion Dollars is a 1km stack of $100 bills
Books of 2023
Even though I did read some fiction last year, non really stuck with me. It appears that I am more interested in non-fiction these days. Strange how these things go.
Quest for Performance
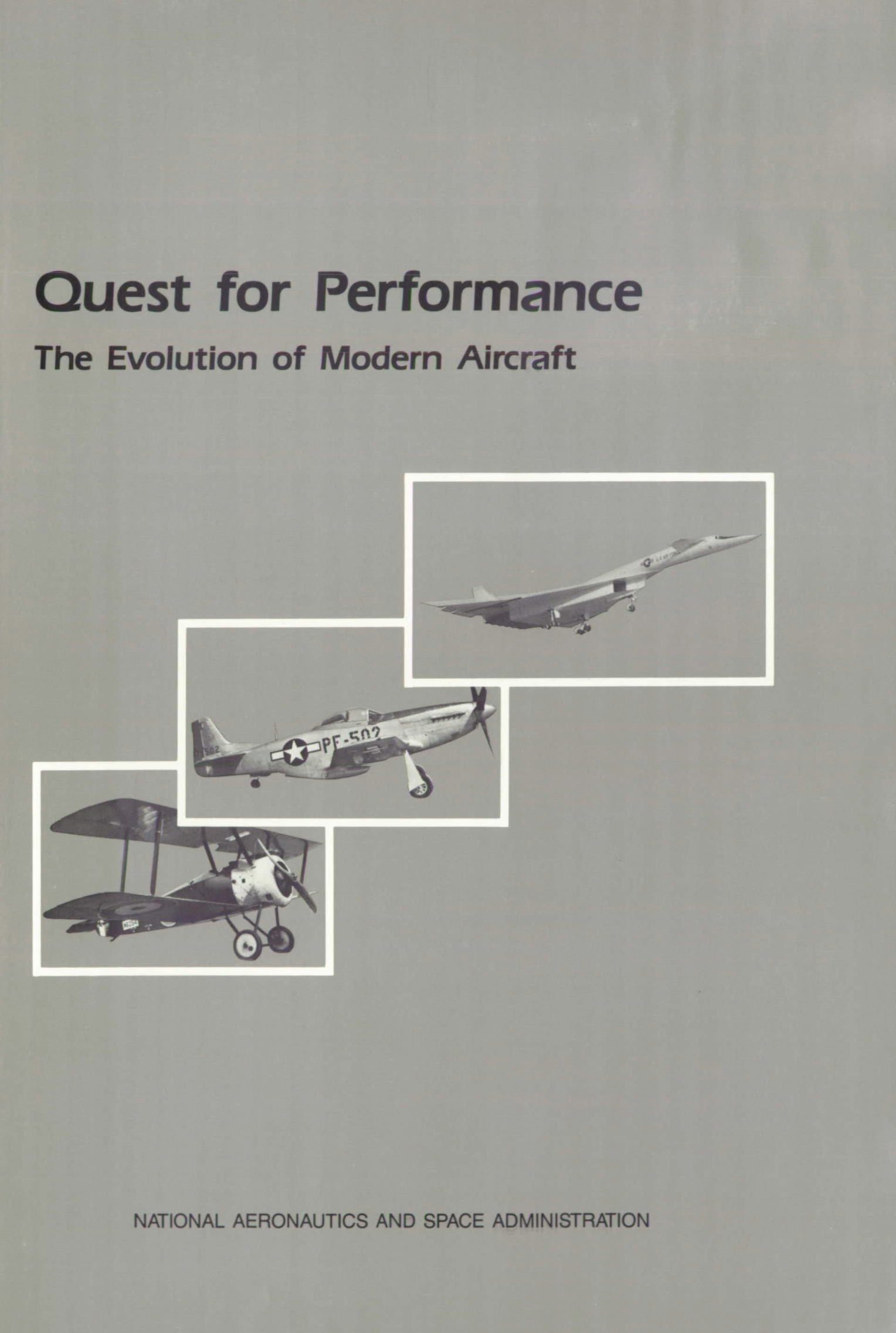
Quest for Performance: The Evolution of Modern Aircraft, by Laurence K. Loftin
I have searched for a book like this for a long time: a history of airplane technology. The book details technological milestones and archetypes from the Wright flyer to the mid-1980s, with an emphasis on the two world wars and interwar years. It sometimes veers too close to a mere list of models and performances, but by and large still manages to tie it all into a comprehensible narrative. I guess you need to be a bit of an airplane nerd to appreciate this, but I found it fascinating!
And it is free to download, too.
The Soul of a New Machine
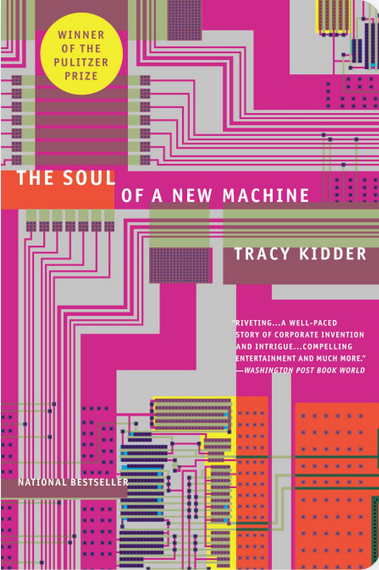
The Soul of a New Machine, by Tracy Kidder
The book retells the development of a computer during the interstitial years, after the big bang of computing in the first half of the century, but before the home computer revolution. This is a bit of a gap in the common computing lore, and one I hadn't know much about.
This happened before standardized CPU architectures, so we get a glimpse into CPU hardware design, the user-land software side of things, and the micro-code in between. This is quite an unusual perspective today, reliant on common abstractions as we are.
A fascinating read if you're interested in computing history, without requiring a Computer Science degree for the broader story.
Die großen Zeppeline
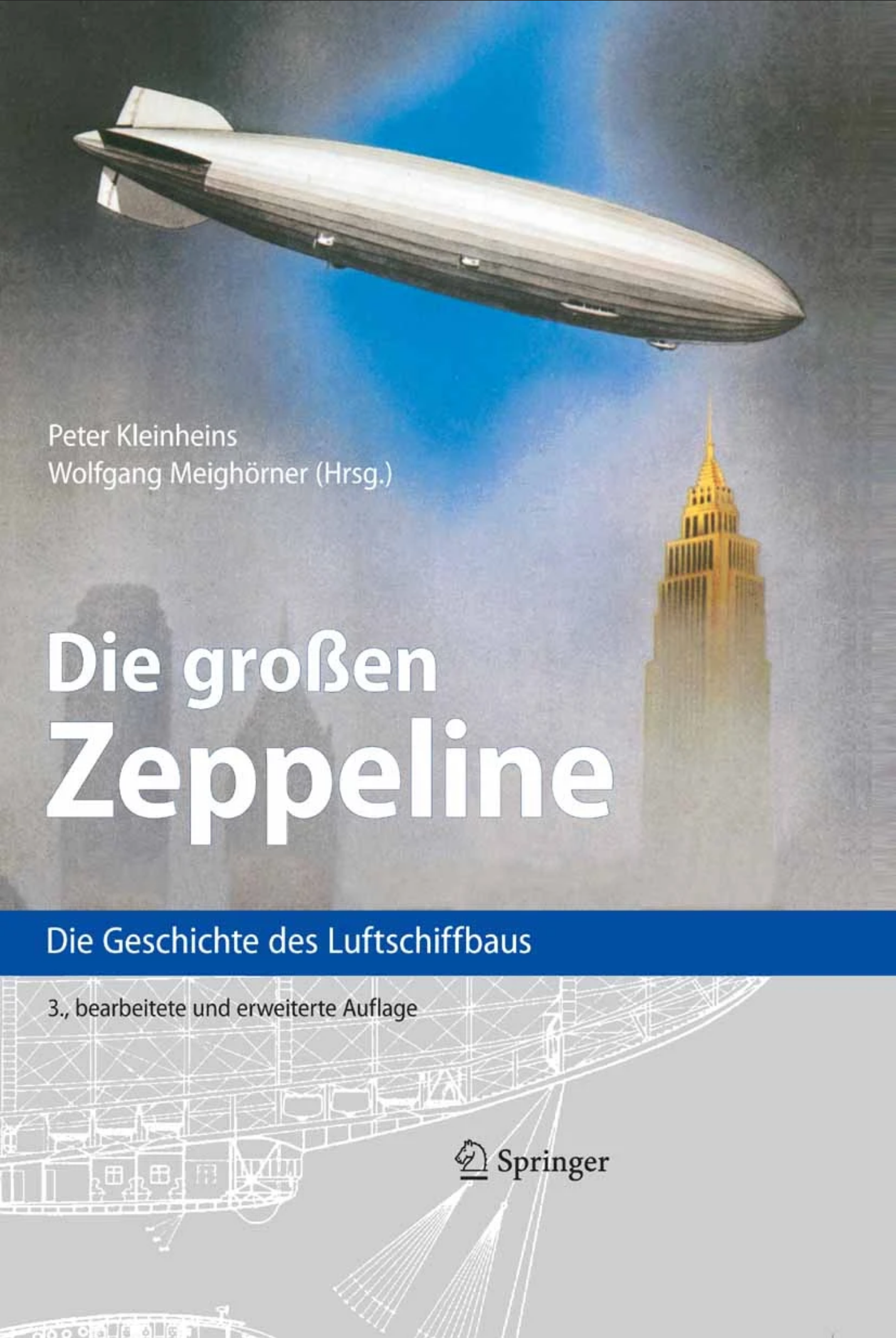
Die großen Zeppeline: Die Geschichte des Luftschiffbaus, by Peter Kleinheins
Half the book is reprints of technical reports of the original lead engineers who worked on the German Zeppelins. The other half is a retrospective view of Zeppelins in Germany and elsewhere.
There are myriad fascinating details about Zeppelin construction, like how their gas bags were made from animal intestines, or how they reclaimed water from engine exhaust to prevent losing weight while burning fuel. And it's especially fascinating to read about these things from people to whom this was the pinnacle of technology, and juxtapose our modern perspective.
This is another book I've been searching for many years. I found both this and Quest for Performance on Library Genesis, which is a terrific resource for researching books.
🪦 Emacs 2011-2023
For the last dozen years, I have used Emacs as my text editor and development environment. But this era ended. In this post, I outline how I went from using Emacs as a cornerstone of my digital life, to abandoning it.
In an ironic twist of history, it was Visual Studio that drove me to Emacs in the first place, and what ultimately pulled me away from it: In 2011, I was working on the firmware of a digital mixing console. This was edited in Visual Studio, compiled with an embedded compiler software, and source-controlled with command-line Git. It was ultimately Emacs that allowed me to tie this hodgepodge of idiosyncratic C+1, Git, and the proprietary compiler into a somewhat sane development environment.
Over the years, my Emacs config grew, I learned Elisp, I published my own Emacs packages, and developed my own Emacs theme. I went back to university, did my PhD, worked both OSS and commercially, and almost all of this was done in Emacs. As particular standouts beyond traditional text editing, I used Emacs' Git-client Magit every single day, and my own org-journal was absolutely vital as my research/work journal.
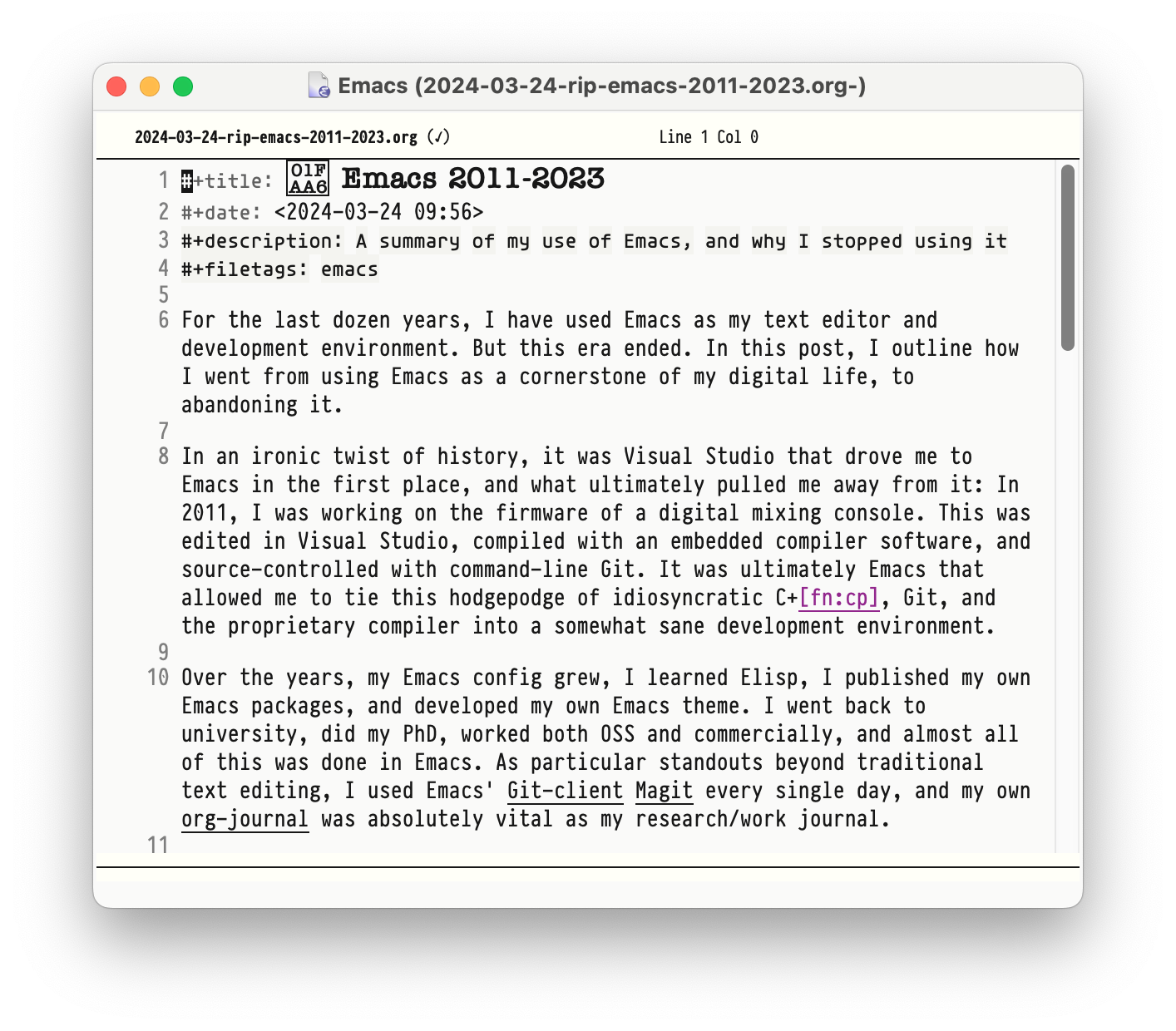
In 2023, however, I started a new job, once again with a Visual Studio codebase. This time, however, the code base and build system was tightly woven into the Visual Studio IDE, and only really navigable and editable therein. It thus made no sense to edit this code in Emacs, so I didn't. Perhaps I also needed a break.
And as my Emacs usage waned, so its ancient keyboard shortcuts started to become a liability. I started mis-typing Emacs things in Visual Studio, and hitting Windows shortcuts in Emacs. Friction began to arise. At the same time, I started noticing how poorly Emacs runs on Windows. Startup takes many seconds, it does not integrate well into the task bar2, it doesn't handle resolution changes gracefully, and it's best I don't start talking about its horrendously broken mouse scrolling. And of course it can't scroll point out of the window3.
My last use-case for Emacs was org-journal. I ended up porting a basic version of it to Visual Studio Code. Having thus written a text editor plugin for both editors, I have to be blunt: both, the anachronistic bone-headedness of Elisp, and the utter insanity of TypeScript's node APIs, are terrible environments for writing plugins. A few years ago I did the same exercise in Sublime Text Python, which was a beautiful, simple, quick affair. But I do enjoy a programming puzzle, so here we are.
The final nail in Emacs' coffin came from an unexpected corner: For all my professional life, I was a solo coder. My Emacs was proudly black-and-white (different fonts instead of different colors!), and my keyboard shortcuts were idiosyncratically my own. I did not merely use Emacs. I had built MY OWN Emacs. I like to think this built character, and API design experience. But it was of course a complete non-starter for pair programming. After having tasted Visual Studio (± Code) Live Sharing, there was simply no going back.
And thus, I am saddened to see that I haven't started Emacs in several weeks. I guess this is goodbye. This blog is still rendered by Emacs, and I still maintain various Emacs modules. My journal is still written in org-mode. But it is now edited in Visual Studio Code.
Footnotes:
An eclectic subset of C++, intersected with the limitations of the embedded compiler. This was decidedly pre-"modern" C++, and probably less than the sum of its parts.
Usually, the program's taskbar button starts the program, and represents it while running. Emacs spawns a new button instead.
This is emacs-speak for "it can't scroll the cursor outside the viewport"
Two Years with Legacy Code
From January 2021 to the beginning of 2023, I worked on a legacy code base at Fraunhofer IDMT in Oldenburg. My task was the maintenance and development of a DNN-based speech recognition engine that had become terra incognita when its original developer had left the company a year before I started. The code had all the hallmarks of severe technical debt, with layers of half-used abstractions, many unused branches of unknown utility, and the handwriting of several concurrent programmers at odds with each other.
The code had evidently been written in a mad dash to bring the product to market. And not to discredit its developers, had been in production for several years, with a core of robust algorithms surrounded by helper scripts that had allowed the company to build upon, even after the original developers had left.
It was my job to clean it up. Having spent six years on my PhD recently, I welcomed the calmer waters of 'just' programming for a bit. This blog post is a summary of the sorts of challenges I faced during this time, and what kinds of techniques helped me overcome them.
The lay of the land
I approached the task from the outside, sorting through the build scripts first. Evidently, at least three authors were involved: One old-school Unix geek that wrote an outdated dialect of CMake, one high-level Python scripter, and one shell scripter that deeply believed in abstraction-by-information-hiding. The result of this was… interesting.
For a good few weeks I "disassembled" these scripts by tracing their execution manually through their many layers, and writing down the necessary steps that were actually executed. My favorite piece of code was a Makefile that called a shell script that ran a Python program, which instantiated a few classes and data structures, which ultimately executed "configure; make; make install" on another underying Makefile. I derived great satisfaction from cutting out all of these middle-men, and consolidating several directories of scripts into a single Makefile.
Similar simplifactions were implemented at the same time across several code bases by my colleagues. In due time, this concerted effort enabled us to implement continuous integration, automated benchmarking, and automated builds, but more on that later.
Data refactoring
The speech recognition software implemented a sort of interpreter for the DNN layers, originally encoded as a custom binary blob. Apparently, a custom binary approach had been taken to avoid dependencies on external parsing libraries. Yet the data had become so convoluted that both its compilation and its parsing were now considered unchangeable black boxes that impeded further development.
Again, I traced through the execution of the compiling code, noted down the pieces of data it recorded, and rewrote the compiler to produce a MsgPack file. On the parsing side, I wrote a custom MsgPack parser in C. Looking back, every job I've had involved writing at least a couple of data dumpers/parsers, yet many developers seem intimidated by such tasks. But why write such a thing yourself instead of using an off-the-shelf solution? In an unrelated code review later in the year one colleague used the cJSON library for parsing JSON; in the event, cJSON was several magnitudes bigger and more complex than the code base it was serving, which is clearly absurd. Our job as developers is to manage complexity, including that of our dependencies. In cases such as these, I often find a simple, fit-for-purpose solution preferable to more generalized external libraries.
A part of the DNN data came from the output of a training program. This output however was eternally unstable, often breaking unpredictably between version, and requiring complex workarounds to accommodate different versions of the program. The previous solution to this was a deeply nested decision tree for the various permutations the data could take. I simplified this code tremendously by calling directly into the other program's libraries, instead of trying to make sense of its output. This is another technique I had to rely on several times, hooking into C/C++ libraries from various Python scripts to bridge between data in a polyglot environment.
Doing these deep dives into data structures often revealed unintended entanglements. In order to assemble one data structure, you had to grab pieces of multiple different source data. Interestingly, once data structures were cleaned up to no longer have such entanglements, algorithms seemed to fall into place effortlessly. However, this was not a one-step process, but instead an ongoing struggle to keep data structures minimal and orthogonal. While algorithms and functions often feel easier to refactor than data structures, I have learned from this that it is often the changes to data structures that have the greatest effect, and should therefore receive the greatest scrutiny.
Code refactoring
My predecessor had left me a few screen casts by way of documentation. While the core program was reasonably well-structured, it was embedded in an architectural curiosity that told the tale of a frustrated high-level programmer forced to do low-level gruntwork. There were poor-man's-classes implemented as C structs with function pointers, there were do-while-with-goto-loops for exception handling, there were sort-of-dynamically-typed data containers, accompanied by angry comments decrying the stupidity of C.
Now I like my high-level-programming as much as the next guy, but forcing C to be something it isn't, is not my idea of fun. So over a few months I slowly removed most of these abstractions. Somewhat to my surprise, most of them turned out pure overhead that could simply be removed. Where a replacement was needed, I reverted to native C constructs. Tagged unions instead of casting, variable-length-arrays instead of dynamic arrays. Treating structs as values instead of references. This, alone, reduced the entire code base by a good 10%. The harder part was sorting out the jumble of headers and dependencies that had evidentally built up over time. Together with the removal of dead code paths, the overall code base shrank by almost half. There are few things more satisfying than excising and deleting unnecessary code.
I stumbled upon one particularly interesting problem when trying to integrate another code base into ours. Within our own software, build times were small enough to make logging and printf-debugging easier than an interactive debugger such as GDB. The other code base however was too complex to recompile on a whim, and a different solution had to be found. Now I am a weird person who likes to touch the raw command line instead of an IDE. And in this case this turned out to be a huge blessing, as I found that GDB can not only be used interactively, but can also be scripted! So instead of putting logging into the other library, I wrote GDB scripts that augmented break points with a little call printf(...)
or print/d X
. These could get suprisingly complicated, where one breakpoint might enable or disable other breakpoints conditionally, and break point conditions could call functions on their own. It took some learning, but these debugging scripts were incredibly powerful, and a technique I will definitely refer to in the future.
When adding new features to the software, I often found it impossible to work the required data flow into the existing program code without snowballing complexity. I usually took these situations as code smells that called for a refactoring. Invariably, each cleaning up of program flow or data structures inched the program closer and closer to allow my feature addition. After a while, this became an established modus operandi: independently clean the code until feature additions become easy and obvious, then do the obvious thing. Thus every task I finished also left the surrounding code in a better state. In the end, about 80% of the code base had gotten this treatment, and I strongly believe that this has left the project in a much better state than it was before. To say nothing of the added documentation and tests, of course.
More velocity makes bigger craters
As I slowly shifted from cleanup work to new features, change management became a pressing issue. New features had to be evaluated, existing features had to be tested, and changes had to be documented and downstreamed. Fascinatingly, the continuous integration and evaluation tools we built for this purpose, soon unearthed a number of hidden problems in other parts of the product that we had not been aware of (including that the main task I had been hired to do was less worthwhile than thaught, LOL). That taught us all a valuable lesson about testing, and proving our assertions. That said, I never found bottom-level unit tests all that useful for our purposes; the truly useful tests invariably were higher-level integration tests.
Eventually, my feature additions led to downstream changes by several other developers. While I took great care to present a stable API, and documenting all changes and behavior appropriately, at the end of the day my changes still amounted to a sizeable chunk of work for others. This was a particularly stark contrast to the previous years of perfect stagnation while nobody had maintained the library. My main objective at this point was to avoid the mess I had started out with, where changes had evidentally piled on changes until the whole lot had become unmaintainable.
Thus a balance had to be struck between moving fast (and breaking things), and projecting stability and dependability. One crucial tool for this job turned out to be code reviews. By involving team members directly with the code in question, they could be made more aware of its constraints and edge cases. It took a few months to truly establish the practice, but by the end of a year everyone had clearly found great value in code reviews as a tool for communication.
Conclusions
There is a lot more to be said about my time at Fraunhofer. The deep dive into the world of DNN engines was truly fascinating, as were the varied challenges of implementing these things on diverse platforms such as high-performance CPU servers, Laptops, Raspberry Pis, and embedded DSPs. I learned to value automation of developer tasks, and of interface stability and documentation for developer productivity.
But most of all, I learned to appreciate legacy code. It would have been easy to call it a "mess", and advocate to rewrite it from scratch. But I found it much more interesting to try to understand the code's heritage, and tease out the algorithmic core from the abstractions and architectural supports. There were many gems to be found this way, and a lot to be learned from the programmers before you. I often felt a strange connection to my predecessor, as if we were talking to each other through this code base. And no doubt my successor feels the same way about my code now.

bastibe.de by Bastian Bechtold is licensed under a Creative Commons Attribution-ShareAlike 3.0 Unported License.